One of the best Technique to RINGS
작성자 정보
-
Aaron 작성
- 작성일
본문
Machine learning (ML) plays an increasingly important role in improving the recycling of tungsten carbide through various applications. Heres a detailed look at how ML can enhance different aspects of the recycling process:
### 1. **Material Sorting and Classification**
- **Automated Sorting**: ML algorithms can analyze data from sensors (like X-ray or near-infrared) to identify and classify materials based on their chemical composition. This enables more accurate and efficient sorting of tungsten carbide from other metals and contaminants.
- **Image Recognition**: Computer vision techniques can be employed to visually inspect materials on conveyor belts, allowing for real-time identification and sorting of tungsten carbide-based products.
### 2. **Predictive Maintenance**
- **Equipment Monitoring**: Machine learning can analyze data from machinery used in the recycling process to predict when maintenance is needed. This helps prevent breakdowns and downtime, leading to more efficient operations.
- **Performance Optimization**: By analyzing historical performance data, ML can identify patterns that indicate optimal operating conditions, enabling adjustments to improve efficiency.
### 3. **Process Optimization**
- **Parameter Tuning**: ML algorithms can be used to optimize various parameters in the recycling process, such as temperature, pressure, and chemical concentrations during the refining stage, to maximize recovery rates and material quality.
- **Flow Optimization**: Machine learning can help streamline the workflow in recycling facilities by analyzing data on material inflow, processing times, and output quality, leading to improved overall efficiency.
### 4. **Quality Control**
- **Real-Time Monitoring**: ML models can monitor the quality of recycled tungsten carbide in real time, detecting deviations from desired specifications. This allows for immediate adjustments to maintain quality standards.
- **Defect Detection**: Advanced algorithms can identify defects or inconsistencies in the recycled material, ensuring that only high-quality products are produced.
### 5. **Supply Chain Management**
- **Demand Forecasting**: Machine learning can analyze market trends and historical data to predict demand for recycled tungsten carbide, helping companies optimize inventory levels and custom rings production schedules.
- **Resource Allocation**: ML algorithms can assist in determining the most efficient allocation of resources across the recycling process, improving overall productivity.
### 6. **Data-Driven Decision Making**
- **Insights and Reporting**: Machine learning can synthesize large volumes of data from various sources (e.g., operational data, market trends) to provide insights that inform strategic decision-making.
- **Identifying Trends**: By analyzing patterns in recycling processes, ML can help identify areas for improvement, reduce waste, and enhance the economic viability of recycling operations.
### Conclusion
The integration of machine learning into the tungsten carbide recycling process offers numerous benefits, including enhanced sorting accuracy, optimized operations, improved quality control, and better supply chain management. As technology advances, the role of machine learning in recycling is expected to grow, contributing to more efficient and sustainable practices in the industry.
Abalone Tungsten Ring with Opal Center 8mm - Carbide CUSTOM MADE Engraved Men Women | Wedding bands
Machine learning (ML) plays an increasingly important role in improving the recycling of tungsten carbide through various applications. Heres a detailed look at how ML can enhance different aspects of the recycling process:
### 1. **Material Sorting and Classification**
- **Automated Sorting**: ML algorithms can analyze data from sensors (like X-ray or near-infrared) to identify and classify materials based on their chemical composition. This enables more accurate and efficient sorting of tungsten carbide from other metals and contaminants.
- **Image Recognition**: Computer vision techniques can be employed to visually inspect materials on conveyor belts, allowing for real-time identification and sorting of tungsten carbide-based products.
### 2. **Predictive Maintenance**
- **Equipment Monitoring**: Machine learning can analyze data from machinery used in the recycling process to predict when maintenance is needed. This helps prevent breakdowns and downtime, leading to more efficient operations.
- **Performance Optimization**: By analyzing historical performance data, ML can identify patterns that indicate optimal operating conditions, enabling adjustments to improve efficiency.
### 3. **Process Optimization**
- **Parameter Tuning**: ML algorithms can be used to optimize various parameters in the recycling process, such as temperature, pressure, and chemical concentrations during the refining stage, to maximize recovery rates and material quality.
- **Flow Optimization**: Machine learning can help streamline the workflow in recycling facilities by analyzing data on material inflow, processing times, and output quality, leading to improved overall efficiency.
### 4. **Quality Control**
- **Real-Time Monitoring**: ML models can monitor the quality of recycled tungsten carbide in real time, detecting deviations from desired specifications. This allows for immediate adjustments to maintain quality standards.
- **Defect Detection**: Advanced algorithms can identify defects or inconsistencies in the recycled material, ensuring that only high-quality products are produced.
### 5. **Supply Chain Management**
- **Demand Forecasting**: Machine learning can analyze market trends and historical data to predict demand for recycled tungsten carbide, helping companies optimize inventory levels and production schedules.
- **Resource Allocation**: ML algorithms can assist in determining the most efficient allocation of resources across the recycling process, improving overall productivity.
### 6. **Data-Driven Decision Making**
- **Insights and Reporting**: Machine learning can synthesize large volumes of data from various sources (e.g., operational data, market trends) to provide insights that inform strategic decision-making.
- **Identifying Trends**: By analyzing patterns in recycling processes, ML can help identify areas for improvement, reduce waste, and enhance the economic viability of recycling operations.
### Conclusion
The integration of machine learning into the tungsten carbide recycling process offers numerous benefits, including enhanced sorting accuracy, optimized operations, improved quality control, and better supply chain management. As technology advances, the role of machine learning in recycling is expected to grow, contributing to more efficient and sustainable practices in the industry.
Gold Classic Tungsten Ring 4mm - Carbide CUSTOM MADE Engraved Men Women | Wedding bands
### 1. **Material Sorting and Classification**
- **Automated Sorting**: ML algorithms can analyze data from sensors (like X-ray or near-infrared) to identify and classify materials based on their chemical composition. This enables more accurate and efficient sorting of tungsten carbide from other metals and contaminants.
- **Image Recognition**: Computer vision techniques can be employed to visually inspect materials on conveyor belts, allowing for real-time identification and sorting of tungsten carbide-based products.
### 2. **Predictive Maintenance**
- **Equipment Monitoring**: Machine learning can analyze data from machinery used in the recycling process to predict when maintenance is needed. This helps prevent breakdowns and downtime, leading to more efficient operations.
- **Performance Optimization**: By analyzing historical performance data, ML can identify patterns that indicate optimal operating conditions, enabling adjustments to improve efficiency.
### 3. **Process Optimization**
- **Parameter Tuning**: ML algorithms can be used to optimize various parameters in the recycling process, such as temperature, pressure, and chemical concentrations during the refining stage, to maximize recovery rates and material quality.
- **Flow Optimization**: Machine learning can help streamline the workflow in recycling facilities by analyzing data on material inflow, processing times, and output quality, leading to improved overall efficiency.
### 4. **Quality Control**
- **Real-Time Monitoring**: ML models can monitor the quality of recycled tungsten carbide in real time, detecting deviations from desired specifications. This allows for immediate adjustments to maintain quality standards.
- **Defect Detection**: Advanced algorithms can identify defects or inconsistencies in the recycled material, ensuring that only high-quality products are produced.
### 5. **Supply Chain Management**
- **Demand Forecasting**: Machine learning can analyze market trends and historical data to predict demand for recycled tungsten carbide, helping companies optimize inventory levels and custom rings production schedules.
- **Resource Allocation**: ML algorithms can assist in determining the most efficient allocation of resources across the recycling process, improving overall productivity.
### 6. **Data-Driven Decision Making**
- **Insights and Reporting**: Machine learning can synthesize large volumes of data from various sources (e.g., operational data, market trends) to provide insights that inform strategic decision-making.
- **Identifying Trends**: By analyzing patterns in recycling processes, ML can help identify areas for improvement, reduce waste, and enhance the economic viability of recycling operations.
### Conclusion
The integration of machine learning into the tungsten carbide recycling process offers numerous benefits, including enhanced sorting accuracy, optimized operations, improved quality control, and better supply chain management. As technology advances, the role of machine learning in recycling is expected to grow, contributing to more efficient and sustainable practices in the industry.
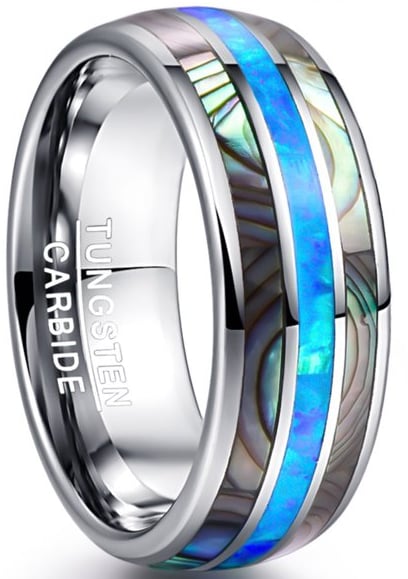
Machine learning (ML) plays an increasingly important role in improving the recycling of tungsten carbide through various applications. Heres a detailed look at how ML can enhance different aspects of the recycling process:
### 1. **Material Sorting and Classification**
- **Automated Sorting**: ML algorithms can analyze data from sensors (like X-ray or near-infrared) to identify and classify materials based on their chemical composition. This enables more accurate and efficient sorting of tungsten carbide from other metals and contaminants.
- **Image Recognition**: Computer vision techniques can be employed to visually inspect materials on conveyor belts, allowing for real-time identification and sorting of tungsten carbide-based products.
### 2. **Predictive Maintenance**
- **Equipment Monitoring**: Machine learning can analyze data from machinery used in the recycling process to predict when maintenance is needed. This helps prevent breakdowns and downtime, leading to more efficient operations.
- **Performance Optimization**: By analyzing historical performance data, ML can identify patterns that indicate optimal operating conditions, enabling adjustments to improve efficiency.
### 3. **Process Optimization**
- **Parameter Tuning**: ML algorithms can be used to optimize various parameters in the recycling process, such as temperature, pressure, and chemical concentrations during the refining stage, to maximize recovery rates and material quality.
- **Flow Optimization**: Machine learning can help streamline the workflow in recycling facilities by analyzing data on material inflow, processing times, and output quality, leading to improved overall efficiency.
### 4. **Quality Control**
- **Real-Time Monitoring**: ML models can monitor the quality of recycled tungsten carbide in real time, detecting deviations from desired specifications. This allows for immediate adjustments to maintain quality standards.
- **Defect Detection**: Advanced algorithms can identify defects or inconsistencies in the recycled material, ensuring that only high-quality products are produced.
### 5. **Supply Chain Management**
- **Demand Forecasting**: Machine learning can analyze market trends and historical data to predict demand for recycled tungsten carbide, helping companies optimize inventory levels and production schedules.
- **Resource Allocation**: ML algorithms can assist in determining the most efficient allocation of resources across the recycling process, improving overall productivity.
### 6. **Data-Driven Decision Making**
- **Insights and Reporting**: Machine learning can synthesize large volumes of data from various sources (e.g., operational data, market trends) to provide insights that inform strategic decision-making.
- **Identifying Trends**: By analyzing patterns in recycling processes, ML can help identify areas for improvement, reduce waste, and enhance the economic viability of recycling operations.
### Conclusion
The integration of machine learning into the tungsten carbide recycling process offers numerous benefits, including enhanced sorting accuracy, optimized operations, improved quality control, and better supply chain management. As technology advances, the role of machine learning in recycling is expected to grow, contributing to more efficient and sustainable practices in the industry.
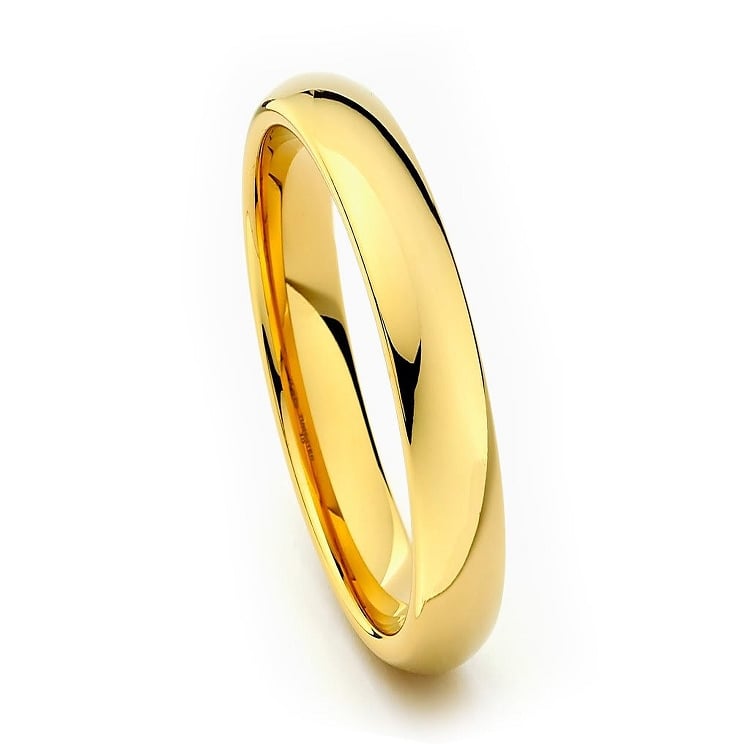
관련자료
-
이전
-
다음
댓글 0
등록된 댓글이 없습니다.